Multi-task learning could be a machine learning paradigm that improves the performance of every task by exploiting useful information contained in multiple related tasks. Data poisoning is an important tool. The security of machine learning algorithms has become a great concern in many real-world applications involving adversaries. The causative attacks where attackers manipulate training examples to subvert the learned model.
Poisoning attacks usually occur when we collect training data from public sources. Data poisoning is an important tool that is very harmful because of its long-lasting effect on the learned model. Also, it is critical for improving the robustness of real-world machine learning systems.
Logic corruption
It is that the foremost dangerous scenario. Logic corruption happens when the attacker can change the algorithm and also the way it learns. At this stage the machine learning part stops to matter because the attacker can simply encode any logic they have. You’d possibly when well are employing a bunch of if statements.
Modification
Next up is data modification. One thing they’ll do is manipulate labels. As an example, they’ll randomly draw new labels for a component of the training pool or try to optimize them to cause maximum disruption. Data poisoning is an important tool. The attack is compatible if the goal is availability compromise but becomes tougher if the attacker wants to place in a very backdoor. Such attacks are similar in how they get pleasure from extra information available to the attacker (white box, black box, etc).
Manipulating
Manipulating the input may be a more sophisticated attack not only because it’s more powerful but also because it is a more realistic threat model behind it. Data poisoning is an important tool. It’s easy for the adversary to insert any files they like but they have no control over the labeling process, which is finished either automatically or manually by a person’s on the alternative end.
Data Injection
Data injection is analogous to data manipulation, except, similar to the name suggests, it’s limited to addition. If the attacker is in a very position to inject new data into the training pool that also makes them an awfully powerful adversary.
Transfer Learning
Transfer learning is the weakest level of adversarial access. Further it is the weakest of the four.
Data Poisoning Attacks on MTRL :
Direct Attack
Data poisoning is an important tool. Further attacker can directly inject data to any or all the target tasks. As an example, in product review sentiment analysis, review as negative or positive. On e-commerce platforms like Amazon, attackers can directly attack the target tasks by providing crafted reviews to the target products.
Indirect attack:
For example, personalized recommendations treat each user as a task and use users’ feedback to teach personalized recommendation models. Further in such scenarios, attackers usually cannot access the training data of target tasks. Data poisoning is an important tool. However, attackers can launch indirect attacks by faking some malicious user accounts. It is in a position to treat as attacking tasks, and providing crafted feedback to the systems.
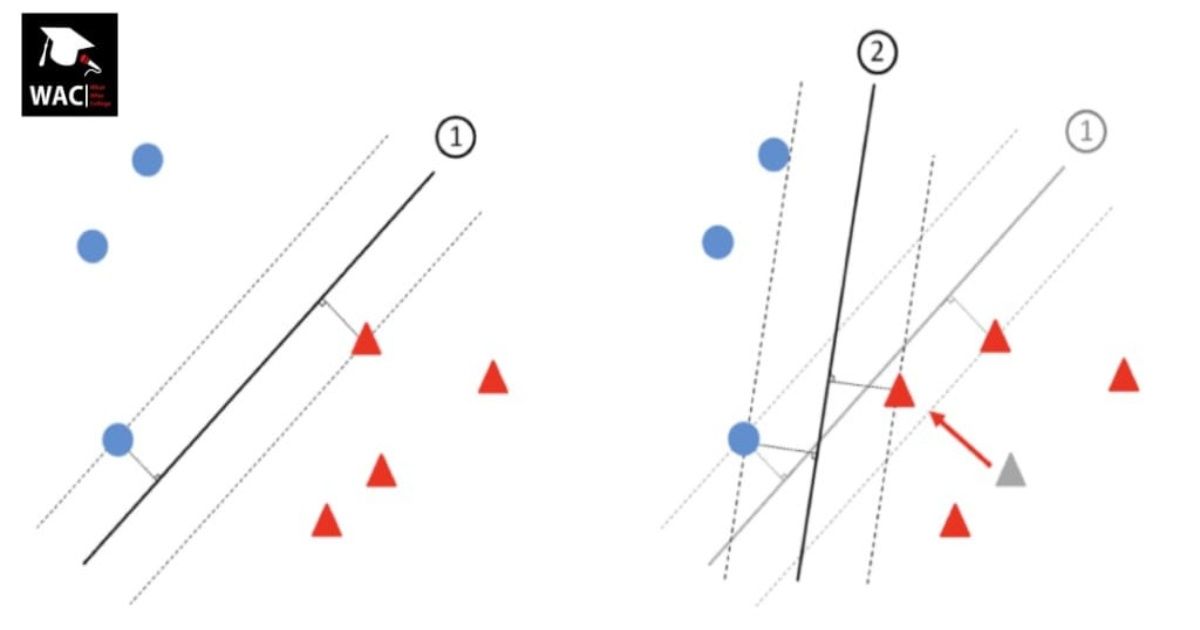
All you need to know about Data Science
Learn Data Science
Top 7 Data Science University/ Colleges in India | Top 7 Training Institutes of Data Science |
Top 7 Online Data Science Training Programs | Top 7 Certification Courses of Data Science |
Learn Data Science with WAC
Data Science Webinars | Data Science Workshops |
Data Science Summer Training | Data Science One-on-One Training |
Data Science Online Summer Training | Data Science Recorded Training |
Other Skills in Demand
Artificial Intelligence | Data Science |
Digital Marketing | Business Analytics |
Big Data | Internet of Things |
Python Programming | Robotics & Embedded System |
Android App Development | Machine Learning |